Team ID: O1-02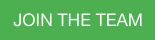
Team Title:
Quantification of Uncertainties in Space Weather Forecasts
Team Lead:
Nikolai Pogorelov (University of Alabama in Huntsville, USA) np0002 AT uah.edu
Team Co-Leads:
Enrico Camporeale (University of Colorado, USA), enrico.camporeale AT colorado.edu
Ming Zhang (Florida Institute of Technology, USA) mzhang AT fit.edu
Keywords (Other):Enter my own keyword (impact):Yes
- CME
- SEP
- uncertainties
Keywords (Activity Type):
Understanding , Modeling, Forecasting , Data Utilization , Assessment
Introduction:
Successful space weather (SWx) forecasts require a synergy of data analysis, numerical simulations, and machine learning (ML). A traditional way to address the challenges in SWx physics is to derive the boundary conditions for coronal models using data assimilation on the photosphere and surface flux transport models, run coronal models to heliocentric distances outside of the Alfvénic surface, and use the obtained solutions in corresponding heliospheric models. Because of the uncertainties in the photospheric boundary conditions, ensemble modeling is inevitable for SWx forecasts. Coronal mass ejections (CMEs) are typically inserted into the ambient solar wind in the corona. This procedure is also characterized by uncertainties in the determination of CME properties from their remote observations. The major challenges in SWx forecasts are the correct determination of CME arrival at Earth (or other planets) and the magnetic field they carry. A plethora of ensemble simulations has demonstrated that uncertainties are not acceptable, being about 12 hours for CME arrival on the average. Recent publications show that ML techniques can be used (1) to automatize the quantification of uncertainties in ensemble modeling and (2) improve the forecasts themselves. For example, a mismatch between the modeled and observed CME arrival can be decreased 2-3 times even if no ensemble performs acceptably. Once a successful forecast is made, it is possible to backtrack the reasons of errors introduced by multiple uncertainties and determine the sensitivity of forecasts to different sources of uncertainties. ML is also of importance for modeling solar energetic particles (SEPs), where uncertainties additionally exist in the process of ion injection into the acceleration process.
Objectives:
We propose a SWAT team to address the above issues. Our objective is to use the synergy of simulations, data analysis, and machine learning to improve space weather forecasts. While quantifying uncertainties in SWx predictions, we will also focus on their dependence on the the input parameters, i.e., identify those parameters that are especially critical for successful space weather forecasts.
Cluster with overlapping topics:
S2: Ambient solar magnetic field, heating and spectral irradiance, H1: Heliospheric magnetic field and solar wind, H2: CME structure, evolution and propagation through heliosphere, H3: Radiation Environment in Heliosphere, H4: Solar System and beyond
Link to external website: